When your job search drags on for weeks and you feel no closer to landing a job than when you first started, it's easy to get discouraged. But even if you aren't getting the callbacks you were hoping for, now is not the time to call it quits. To stay motivated and focused during this frustrating time, use these six job-search strategies to regain and maintain your momentum.
1. Treat the search like a job
Unemployment often leads to an aimless feeling. The lack of a routine is a major reason your motivation may be waning, as it's a constant reminder of your situation. The key is to treat your search like a real job. Wake up at a reasonable hour and get dressed. Create a schedule with set times for phone calls, emails, social networking and job board searches. Make to-do lists and check off each item as you complete it. After you've completed your to-do list for the day, "clock out" and take part in any leisure activities you enjoy.
In other words, conduct yourself as if a boss were looking over your shoulder. Stay focused on your daily tasks and avoid playing a quick game of Solitaire or Candy Crush when you're supposed to be working. Little indulgences may seem like some of the few perks of unemployment, but they can lead to listlessness and a dip in job-search momentum.
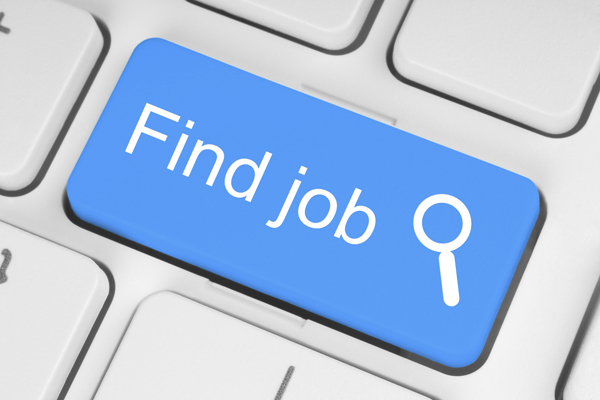
2. Put yourself out there
As important as it is for you to be connected online, you also need to make sure you're occasionally leaving the house. Not only will this help you get out of a rut, but it can also help make you more marketable. Sign up for a class or go to job fairs, workshops, conferences and seminars, where you can meet people and brush up on your skills. Join professional associations and attend their meetings, where you can learn about trends in your field. Volunteer your time and skills with a worthwhile organization, where you can work on your soft skills like written and verbal communications. All of these things will deepen your network and help you find the right job.
3. Be proactive
Don't wait for opportunity to knock. Instead, take the initiative and knock on opportunity's door. In other words, even if the companies you're interested in don't list any current job openings, contact them anyway and express your desire to work there. This extra effort demonstrates enthusiasm and initiative, and hiring managers may take notice.
4. Track your progress
When you start to feel like you're going nowhere, take some time to create a method to track the efforts you've made. Write up a list of realistic short- and long-term goals with regard to your job search, and work toward them every day. For example, decide how many applications you'd like to send out this week, or this month. Set a goal for the number of networking events you're going to attend, and for the number of new people you're going to talk to about your search. Then keep track as you move toward the goal. That way, you'll have a tangible way to prove to yourself that you've made progress, something that can help keep you motivated as you continue to look for a job.
5. Consider other work options
A full-time job with a check direct-deposited to your tài khoản is not the only type of work out there. You can also expand your search to include part-time and contract work or set yourself up as a consultant or freelancer. Maybe you can barter your skills in exchange for goods and services.
Signing up with a staffing agency for temporary or project-based gigs can also be a productive approach. It can bring in extra income while you're looking for full-time work. Even better, some part-time or temporary gigs can turn into full-time jobs or long-term contracts. Even if they don't, though, they'll still allow you to make valuable contacts that will help you in your job search.
6. Relax, recharge, revive
Allowing a job search to take over your life is a sure way to burn out. Give yourself permission to take a break from the search at night and on weekends. When you make a point to relax and recharge for a few hours at the end of the work day, you'll be able to start fresh the next day. A change of scenery and new experiences may give you a new perspective on your search and even your career.
The key to finding employment is to keep at it. Don't let a lull discourage you to the point of giving up. By following these job-search tips and persevering, you'll greatly increase your chances of finding full-time work that is satisfying and rewarding.
(Picture Source: Internet)
HRVietnam - Collected
Teaching Machines to Think About HR
For all its promise for HR, big data and its “machine-learning” component still only give us facts about, and factual relationships within, our workforces; not conclusions based on the statistical analyses HR has always needed—and always will—to make meaningful predictions.
By Peter Cappelli
Workforce analytics. Big data. Machine learning.
The above terms—or “buzzwords” if you don’t like them—are popping up in many discussions of human resources, mainly involving vendors with solutions that make use of new data aimed at answering traditional workforce questions. Is there anything really new in these approaches, and, if there is, should we be paying attention to it?
The answers are yes and yes.
Let’s start with the term workforce analytics. In some ways, this term is to traditional measures of outcomes as human resources was to personnel. It is about addressing traditional, evergreen questions in different, more sophisticated ways.
Workforce analytics describes an effort to use data and sophisticated analyses to address HR problems. The most topical ones at the moment are: “Which candidates will make the best hires?” and “Which employees are most likely to leave?”
There is nothing new about those questions, and there isn’t much new about how they are being approached. The novelty comes, in part, from the fact that, after the early 1980s, big corporations gave up trying to address these questions in a sophisticated manner, so most people in business aren’t aware that similar approaches were tried a generation or so ago.
But there are some differences. One is a greater interest in analyses pertaining to financial outcomes: e.G., It will save us $5,000 per employee by reducing turnover.
The second difference involves the type of data available. The “Manplan” program in the 1960s required HR staff to read information about an employee from one file, mechanically punch it onto a card, then get different information for that same employee from another file, punch it onto a different card, then do that for every employee they wanted to study. Only when those steps were completed could they start looking to see what factors predicted turnover. It cost a fortune to look at even a small set of employees.
Virtually all HR data now is kept electronically, and, in most companies, the information on every applicant who tried to get a job with them is sitting somewhere in a dataset. It’s much easier and cheaper to look at huge numbers of observations, which makes it much easier to find potentially useful results. Being able to capture every “hit” on your employee-benefits website, for example, can tell us almost instantly what kinds of employees are worried about what types of issues.
But here’s the brake to the Big Data bandwagon: Not all HR data is big. The key piece of information needed to make workforce analytics valuable is a measure of job performance. We can’t say anything about which of our 1 million applicants will do well without being able to identify who among our employees is a good performer. In most companies, that information is no better than it was in the 1950s, and, in many companies, it is actually worse, as we’ve gone from assessment-center scores to a supervisor’s guess about potential. The phrase “garbage in/garbage out” is highly relevant here.
That takes us to the last and most obtuse of the buzzwords: machine learning.
It is a different way to think about data than most of us have previously seen, one that came from people whose expertise was rooted in computers rather than statistics per se. The “learning” idea comes from the fact that computer programs (i.E., The “machine”) can be designed to look at data and find patterns that allow them to make predictions.
How exactly machine learning differs from statistics is a topic of endless fascination to people in those two fields, but for the rest of us, here’s what matters: The traditional, statistical approach to analyzing HR data begins with hypotheses that come from prior research. It includes careful statements about assumptions in the study, or studies, and whether those assumptions are true. Traditional machine learning, in contrast, is theory-free and assumption-free. It just looks for patterns in the data, and it uses different techniques from what had commonly been used in statistics to find the clearest patterns.
A statistical examination of whether a given employment test predicts good hires concludes with either “yes” or “no,” where "no" means we can’t rule out that the relationships were due to chance.
In the case of a machine-learning examination, an employee might instead conclude that, while there is no overall relationship, there is a very powerful relationship for this subset of employees, nothing much for that subset, and, for a third subset, a strong relationship that was different than that of the first group.
The power of machine learning comes from the fact that it might well find important predictors that we never thought of before because prior theory didn’t include them—e.G., The distance an applicant’s home is from the work location predicts turnover—and wasn’t particularly adept at “mining” through lots of seemingly unrelated data to find predictors.
All that sounds really promising for machine learning, but there are a bunch of things about this approach that we’d better consider pretty carefully before diving in.
The first of these is a reminder that machine learning produces facts, rather than conclusions. It tells us “X is related to Y,” but not why they are related. Without hypothesis testing and clear statements of assumptions, we don’t learn much about what a given relationship means or why it exists. Perhaps most important, machine learning can’t tell us much about the likelihood that a relationship observed in this dataset will be useful in another context.
This matters in HR because most of the frameworks that support modern employment—especially legal frameworks—relied on the scientific method and traditional statistical tests for their foundation.
Consider, for example, the legal norm that selection tests should not discriminate and the “Uniform Guidelines of Employee Selection Procedures” put together by psychologists over the past generation to ensure that hiring practices are both valid and don’t discriminate against protected groups. Machine learning, as traditionally practiced, would surely uncover relationships that, if applied to hiring, would violate the law.
Machine learning applied to big data will certainly turn up a lot of interesting facts for workforce analytics to ponder. Transferring those facts to practice, however, is still a big leap. On their own, these “buzzword” approaches won’t get us there.
Peter Cappelli is the George W. Taylor Professor of Management and director of the Center for Human Resources at The Wharton School of the University of Pennsylvania in Philadelphia. His latest book is Why Good People Can't Get Jobs: The Skills Gap and What Companies Can Do About It.
Hreonline.Com/HRE/view/story.Jhtml?id=534358638
0 comments:
Post a Comment